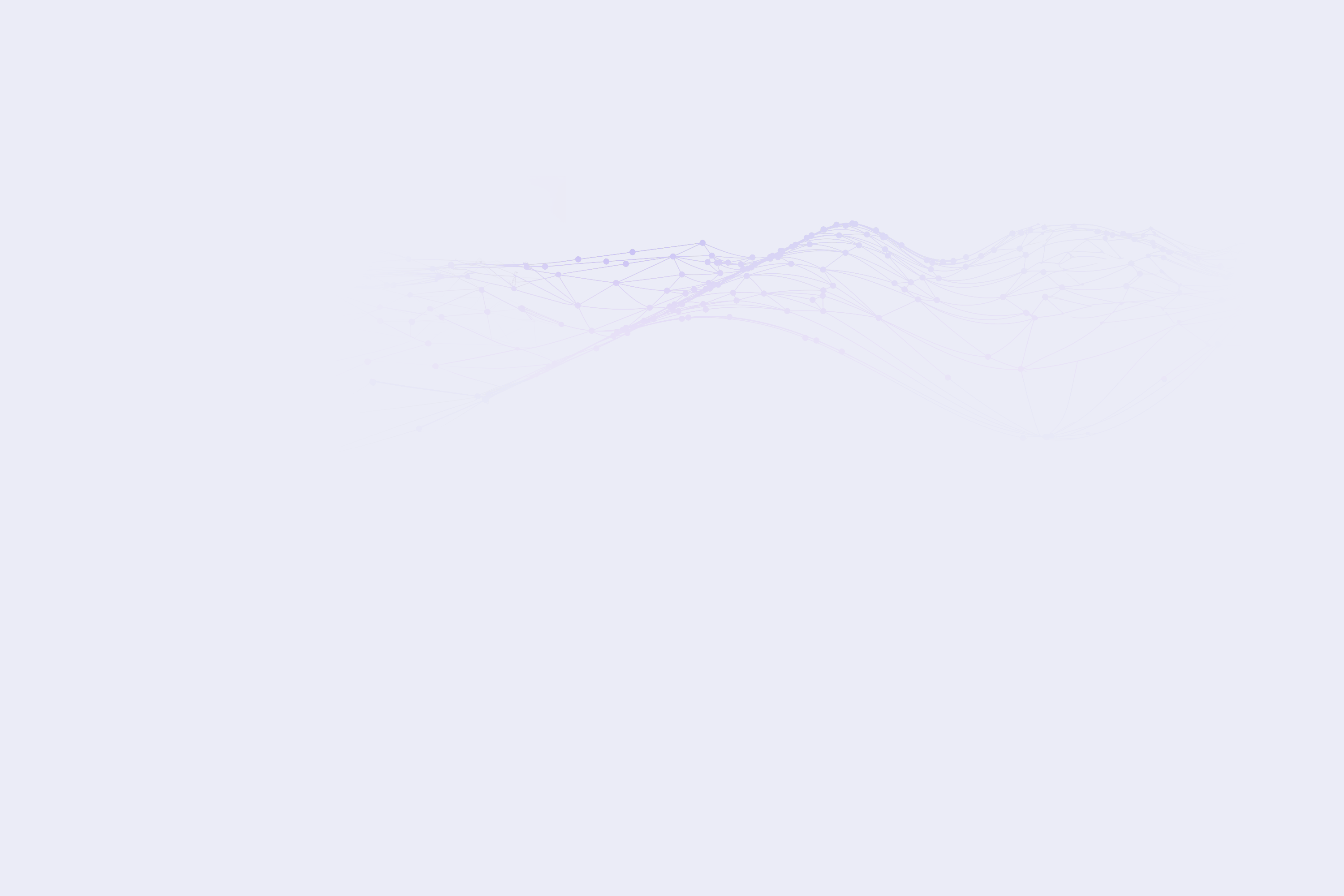
Cifer101
Cifer101 is designed to provide a comprehensive understanding of CiferAI, a cutting-edge technology that integrates blockchain technology with advanced privacy-preserving machine learning frameworks. Tailored for non-technical audiences, this section will help you grasp the core concepts and benefits of CiferAI. Our aim is to show how our solutions address data privacy, security, and decentralized collaboration challenges in AI development, making it easier for you to explore new business opportunities, communicate our technology's value, or consider investing in innovative AI solutions.
What is Cifer?
Cifer is an innovative platform that combines blockchain technology with advanced privacy-preserving machine learning frameworks to address data privacy, security, and decentralized collaboration challenges in AI development.
What Problems Does Cifer Aim to Solve?
Cifer aims to solve key issues related to data privacy, security, and decentralized collaboration in AI development, ensuring that sensitive data remains protected while enabling effective and efficient AI model training and deployment.
What is Decentralized Machine Learning?
Decentralized Machine Learning (DeML) is an approach to AI development where machine learning models are trained across multiple decentralized nodes, rather than a centralized server, enhancing data privacy, security, and collaboration.
What is Privacy-Preserving Machine Learning?
Privacy-Preserving Machine Learning (PPML) involves techniques and methodologies that ensure data privacy and security during the training and deployment of AI models. This includes Federated Learning, Fully Homomorphic Encryption, and Multi-Party Computation, which protect sensitive information from exposure.
How Does Cifer Integrate Decentralized ML and Privacy-Preserving ML?
Cifer integrates decentralized ML and privacy-preserving ML by using blockchain for decentralization and employing advanced privacy-preserving ML frameworks. These frameworks include Federated Learning, Fully Homomorphic Encryption, and Multi-Party Computation, enabling secure, decentralized, and collaborative AI model training.
What is Cifer's Core Product?
Cifer's core product is the Cifer SDK, which is built for machine learning developers. Inside the Cifer SDK, developers have access to a suite of advanced tools and frameworks, including Federated Learning, Fully Homomorphic Encryption, Multi-Agent Systems, Multi-Party Computation, and Swarm Intelligence. These tools enable developers to build secure, privacy-preserving, and decentralized AI models efficiently, facilitating privacy-preserving ML and decentralized ML for secure, private, and collaborative AI development.
What is Federated Learning?
Federated Learning is a machine learning technique where individual devices or servers train models locally on their own data and then aggregate the updates to create a global model. This approach enhances data privacy by ensuring that raw data never leaves the local devices. There are two types of federated learning: centralized and decentralized. In centralized federated learning, a central server coordinates the aggregation of model updates, whereas in decentralized federated learning, devices communicate and aggregate model updates directly with each other without a central server.
What Are the Use Cases for Federated Learning?
Federated Learning has many significant use cases across various sectors. In finance, it is used for credit scoring, personalized banking, and fraud detection, allowing institutions to collaborate on model training without sharing sensitive data. In healthcare, it aids in disease prediction, drug discovery, and treatment planning by enabling medical institutions to jointly develop models while preserving patient privacy. In e-commerce and retail, Federated Learning enhances recommendation systems by aggregating data from different sources to provide personalized shopping experiences without compromising customer privacy.